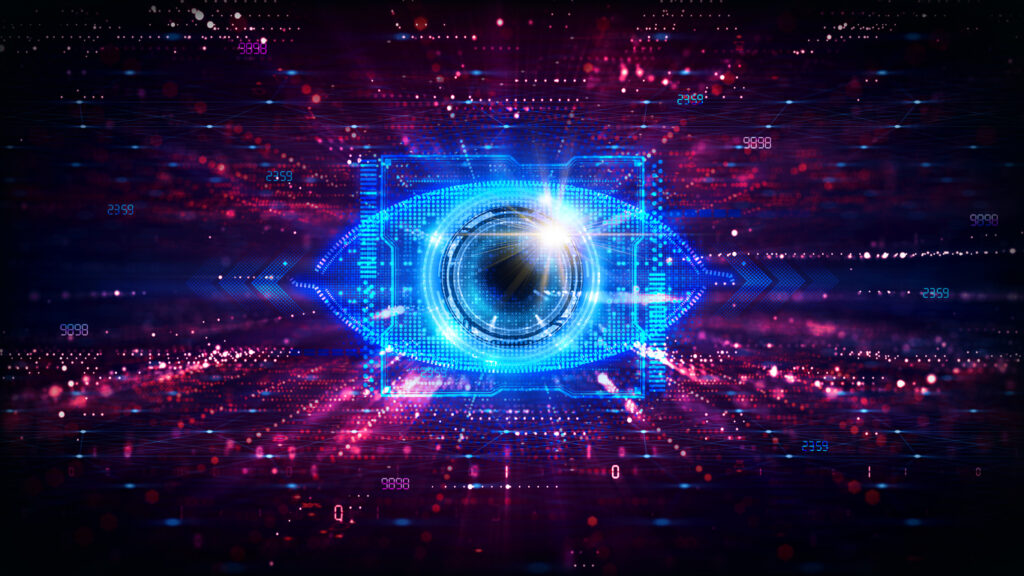
The allure of AI is its ability to store and handle phenomenal amounts of information. However, the amount of data and how it accumulates is a part of the problem. We’ve divided the Downsides of AI into three categories: Privacy, Transparency/Ethics, and Energy Usage.
Privacy
To illustrate this, here is a question to ponder: can a person really hide a medical diagnosis from Big Data? If you were to attempt to do so, what would you do? What would you avoid? A fascinating article on this subject can be found in The Hidden-Pregnancy Experiment, published in May of 2024 in The New Yorker.
We all supply a staggering amount of data via cell phones, wireless technology, car computers that relay information, social media, images shared, internet usage, cameras, and the “Internet of Things” (wireless tech in our homes), etc., and it is almost all fed into algorithms. The data shared per person is enormous and can be invasive regarding the privacy of daily life.
It is not easy to hide a diagnosis. And once the cat is out of the bag, accurately targeted ads appear disturbingly quickly.
Many questions are circulating in IT/Ethics circles about what is considered “personal” and what is not. In a nutshell, the quandary of an individual granting permission for some information to be shared that then can be correlated with other pieces of rather innocent data to then be folded into accurately identifying and/or predicting behaviors or tendencies. This mixture would then be used by AI in say, the hiring process in a surreptitious way. This is where the line is certainly blurred between privacy and transparency.
For an in-depth article on privacy and AI, significant information can be found in this piece written by the Office of the Victorian Information Commissioner (Australia).
Transparency/Ethics
Without realizing it, most of us understand that when a computer performs a task, it is because a human:
1. wrote the code to direct the task and
2. the code includes task-specific parameters
There is something very definitive in the “regular” way computers work.
AI doesn’t have parameters. It learns through various formats and makes its own predictions and conclusions. There is no traceable path from point A (query) to point B (prediction). Considering that AI is based on human knowledge and its inherent biases, this is eyebrow-raising to say the least.
A good article to understand various way biases encoded into AI (or just how AI can actually lie in a staggeringly convincing way) can be found in the Washington Post. It seems as though the Washington Post has taken this situation seriously, as it should, because it was listed as a source although it never published the article listed. Yes, you read that correctly. This situation points out the sheer lack of accountability for mistakes but also, the lack of understanding of how such mistakes are made in the first place.
For additional examples in areas of law enforcement and employment, Technopedia is an excellent source. More situations of AI bias and it causing real-world harm are found in this article, housed at their website. For an all-encompassing look at bias, government and corporate surveillance, lack of regulations governing algorithms, and the way AI is affecting financial credit, college application, employment hiring, Netflix’s documentary, Coded Bias.
Energy Usage
AI is run by data centers and a supply chain that requires a significant amount of energy. By the end of 2022, 326 companies across all sectors bought 77.4 gigawatts of wind and solar power. Tech company contracts added up to about 50% of all clean energy. The top 3 corporate purchasers of wind and solar energy were Amazon, Facebook, and Alphabet (Google’s parent company). While this is impressive, such demand and consolidation in one sector prevents clean energy from helping to power our society in other ways.
So how much clean energy power does that equate to? 18 million homes—which would be almost all the housing in 4 populous states: New Jersey (3.7 million), New York (7.6 million), Pennsylvania (5.7 million), and ⅔ of Connecticut’s housing (1 million of its 1.5 million). Tech companies used half of all of this.
The average ChatGPT query needs nearly 10 times the electricity to process as a Google search. According to a May 2024 article by Goldman Sachs, electricity demand will grow 160% by 2030–fueled by data centers. The corresponding investments in infrastructure is estimated to be about $50 million in the US and over $1 trillion in Europe.
Google is not shy about admitting its energy usage is skyrocketing due to its AI products. In its Annual Environmental Report, the company stated that its emissions in 2023 had risen by 135 when compared with the previous year. Taking 2019 as its base year, Google’s emissions have risen by nearly 50%.
AI is touching many aspects of our lives and will be evolving. An open mind, healthy skepticism, and a demand for accountability (in areas of privacy/transparency, ethics, and environmental ramifications) are the ways to navigate this not-so-new technology as it continues its active transformation of our world.
A List of References Mentioned in this Blog Article:
The Office of the Victorian Information Commissioner (Australia)
ChatGPT Invented a Sexual Harassment Scandal and Named a Real Law Prof as the Accused
8 Times AI Bias Caused Real-World Harm
Coded Bias, documentary
CNBC: Amazon, Meta, and Google Buy More Clean Energy Than Any Other Companies
Goldman Sachs: AI is Poised to Drive 160% Increase in Data Center Power Demand
Google’s Environmental Report 2024
Google’s Emissions Climb Nearly 50% in Five Years Due to AI Energy Demand